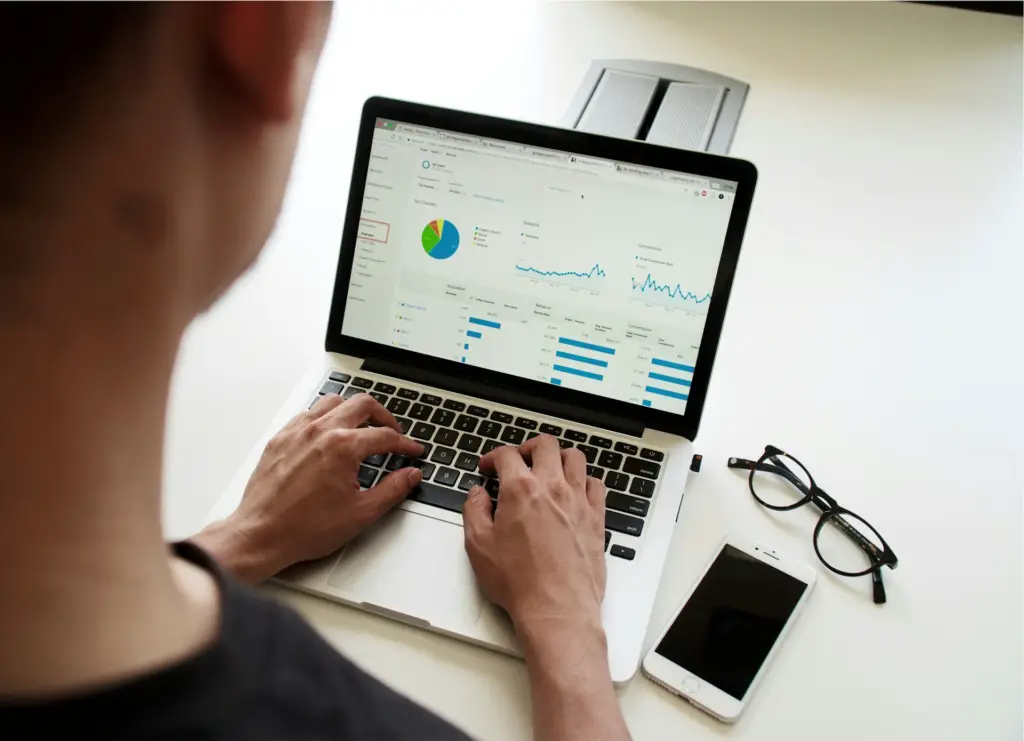
In today’s digital era, organizations in different sectors recognize data as their valuable asset. The ability to effectively collect, manage, and analyze data can give a company an edge. However, the success of any research or analysis depends on the quality of the data being utilized. In this post, we will delve into the significance of data quality in research and analysis for all organizations.
1. Defining Data Quality
Many researchers often wonder, “What is data quality?”. Data quality refers to how data meets certain criteria or requirements. These criteria encompass accuracy, completeness, consistency, reliability, relevance, and timeliness. When it comes to research and analysis, having high-quality data is crucial for generating insights and making informed decisions. Effective data quality management not only enhances the reliability of information but also contributes to building trust in analytical results and supporting strategic decision-making within organizations.
2. Enhancing Accuracy
Accuracy stands out as the most important aspect of data quality. Inaccurate or erroneous data can lead to conclusions and misguided business decisions. For instance, if a marketing team bases its strategies on outdated customer information or incorrect market trends, it could result in wasted resources and missed opportunities.
Maintaining accuracy necessitates monitoring and regular processes for cleaning up data. Addressing duplicates, correcting errors, and ensuring formatting are all steps that enhance the accuracy of your dataset.
3. Ensuring Data Completeness
Having complete data is crucial for gaining an understanding of the subject being studied and conducting a thorough analysis. When data points are missing or incomplete, it can hinder research efforts by limiting insights and distorting patterns that could have been identified with information.
To achieve completeness in datasets, organizations should prioritize thoroughness in their data collection processes. This can be done by implementing question fields and employing validation techniques to minimize entries.
4. Striving for Consistency
Consistency is key when it comes to recording pieces of information across the dataset. Inconsistent data makes it challenging to identify trends or make comparisons between different variables.
To establish consistency in your dataset, consider standardizing measurement units utilizing fill-in options like drop-down lists and adopting naming conventions. Additionally, comprehensive documentation and training within the organization are vital to ensure practices in entering data.
5. Ensuring Reliability
Reliability refers to the trustworthiness and credibility of the data. Reliable data is sourced from credible primary sources and has a proven track record of accuracy over time. Relying on data can undermine research integrity and compromise decision-making processes.
Organizations should invest in data sources while implementing quality control measures during the data collection process. It is crucial to assess the credibility of your data sources and evaluate the quality of information to ensure its reliability.
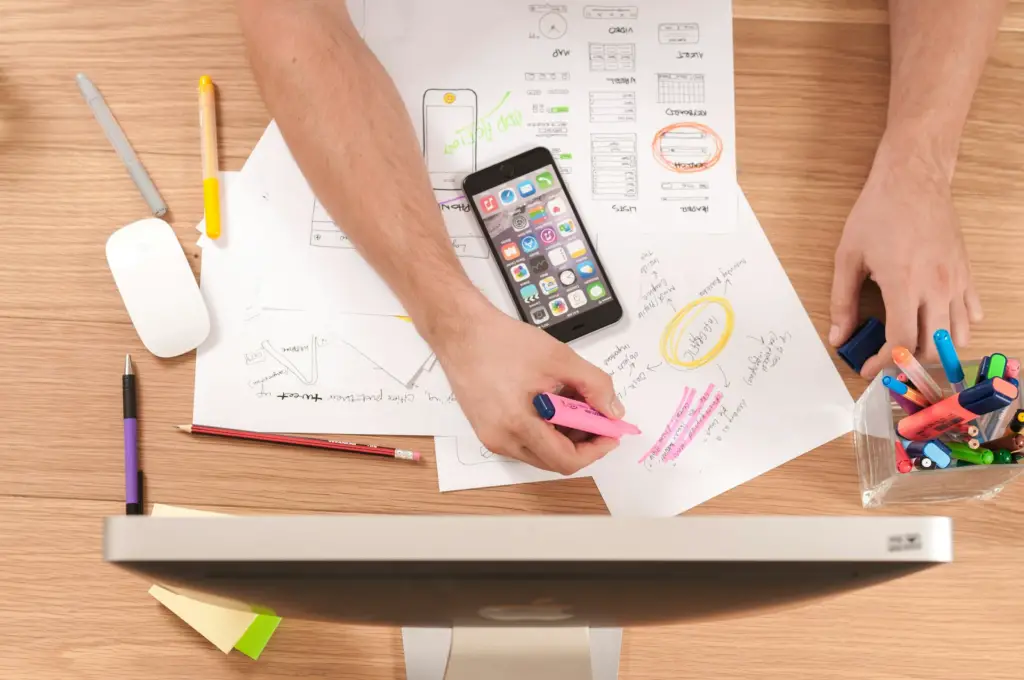
6. The Significance of Relevance
The relevance of data refers to how applicable it is to the objectives of your research or analysis. Having irrelevant or extraneous information can overwhelm analysts, distract from insights, and slow down decision-making processes.
To enhance the relevance of your dataset, it is important to define research objectives and use appropriate filters to screen out irrelevant data. Regularly reviewing and categorizing data based on its relevance will help align it with your organization’s research needs.
7. Embracing Timeliness
Timeliness relates to how up-to-date the collected information is in relation to when it’s needed for analysis or decision-making purposes. Outdated or delayed information significantly diminishes its value in fast-paced industries where real-time insights drive competitive advantages.
Organizations should prioritize timely systems for collecting, validating, and processing data promptly. Implementing checks can help identify time-sensitive information that may negatively impact ongoing projects.
Conclusion
In any organization today, ensuring data quality is an essential aspect of research and analysis.
Companies can gain insights to make decisions by giving importance to accuracy, completeness, consistency, reliability, relevance, and timeliness in their datasets. Furthermore, organizations can position themselves for success by implementing quality control measures, training employees on practices, maintaining high-quality data records, and aligning these efforts with their strategic goals. By understanding the importance of data quality, businesses can unleash their potential and gain a competitive advantage in their respective industries.