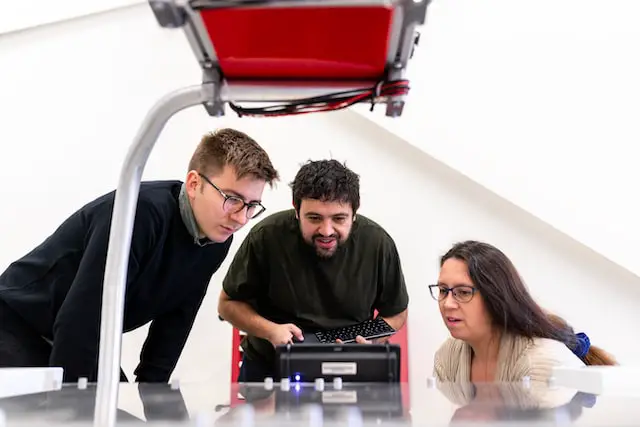
Most people find it challenging to grasp the extent of global money laundering.
The UN estimates that between $800 billion and $2 trillion is laundered yearly.
Thieves transport their money through complex transactions to hide the illegal sources of their revenue. To do this, they need technology that can sift through enormous volumes of data. That is the complexity and scope required of professionals in anti-money laundering (AML).
Using machine learning in AML has become the number one technology for efficiently combating financial crime.
Money Laundering Impact on Financial Institutions
Money laundering and other financial crimes are becoming more commonplace around the globe, and more complex strategies are being utilized to avoid discovery. Banks have responded by increasing their annual investment by many billion dollars to strengthen their barriers against financial crime.
Additionally, as regulators impose harsher penalties, the regulatory fines related to compliance rise year over year. The fight against money laundering is a perennial worry for compliance, monitoring, and risk groups since banks’ conventional rule- and scenario-based methods to combating financial crimes have always looked to be one step behind the bad guys.
Machine Learning and Anti Money Laundering
Criminals frequently use new technologies to get around security system weaknesses because they breach the law. They stand to gain a lot from fraud and money-laundering schemes. Many financial institutions (FIs) may discover that the AML programming capabilities of their outdated legacy systems, many of which are entirely rules-based, are constrained in their efforts to stop money laundering and other illicit actions.
To improve their current rules-based approach, banks and other financial service providers must now revise their anti-money laundering policies and put machine learning models into practice.
Despite this legislation’s noble and significant intentions, financial institutions must deal with constant changes in compliance requirements.
Despite these limitations, money laundering is rising partly due to technology. Megabyte money may now be sent quickly and easily to any location on the earth because to advancements in communications and technology that have led to the creation of an ever-operating global system.
Modern AML systems are built on the principles of machine learning. It builds productive and successful teams by automating case enrichment and prioritizing investigators. Automation reduces the number of false positives, sparing employees from issuing pointless alerts. In contrast, one bank completed the notification procedure in seconds.
Since legacy AML systems are powered by engines that solely apply rules, they frequently produce many low-value warnings. It’s like a crying wolf when a rules-based system generates an absurdly high number of false positives. Analysts analyze a varied number of false-positive alerts each day depending on the size of the bank. Unless you have infinite resources to evaluate signs, you’ll need a different approach. Institutions that failed to spend enough time reviewing high-value warnings produced by automated AML systems have been hit with significant penalties and associated adverse publicity.
Challenges with Traditional AML Methods
Using AML systems that just employ rules has two drawbacks:
- Many false positives are generated when real-time behavior is matched to predetermined criteria. An AML analyst may be required to reply to multiple notifications daily with such a system for transactions that are 100 percent legitimate. That is ineffective because analysts cannot obtain genuine instances, and actual instances are difficult to distinguish from the many cases provided for assessment.
- Money launderers typically change the law themselves. They are consistently behind the offenders by one step. Since the AML system always needs to be updated to consider brand-new illicit activity, analysts are constantly at a disadvantage.
Due to these problems, AML teams that rely entirely on rules run the risk of missing cases of money laundering or missing the deadline for SAR submissions. In all scenarios, the financial institution risks the threat of non-compliance and penalties.
That is how the machine learning models have become an apparent solution in the fight against money laundering. Machine learning models use enormous data sets, and by learning from the actions stored in the data, they may then demonstrate behavior patterns that hint to symptoms of money laundering.
ML Process in AML
Machine learning for AML strengthens models while simultaneously reducing the frequency of high-volume, low-value alerts. That is done so that machine learning models may use algorithms based on historical data to predict future behavior. How models work is as follows:
- Data science teams feed the machine enormous amounts of historical data on instances of known and suspected money laundering.
- Machine learning algorithms create statistical models utilizing the knowledge from these datasets instead of deterministic rules.
- The computer gains knowledge of the characteristics of previous cases of money laundering as well as—and maybe more importantly—what typical behavior comprises.
- The computer projects the likelihood of money laundering instances as well as cases that have been reported to the regulator.
Machine learning can learn and adapt continuously. ML models are only better than the training data you give them. If you can provide the computer-quality, labeled training data to learn from, the computer can learn. As a result, ensure the data sources follow the proper labeling conventions.
Before implementing supervised machine learning models, FIs should assess potential implications on controls, operational processes, and team structure. If your team gains confidence in the performance of the supervised model and regulators show satisfaction with the efficacy of the current controls, your business may consider converting to unsupervised ML models.
These can spot behaviors that significantly deviate from expected patterns. Due to the rules’ limited alert budget under certain circumstances, teams can look into other transactions.
Conclusion
Financial organizations operate in a highly regulated environment, and because anti-money laundering departments have limited tolerance for error, they typically hesitate to be early adopters of new technologies. As a result of their perception that regulators want to see tried-and-true AML practices, many institutions are hesitant to innovate.
To create innovative, market-leading machine learning solutions that enable them to focus more on actually suspicious conduct and send information to law enforcement more rapidly, organizations must overcome these obstacles by working closely with experts who offer ML development services.