Artificial intelligence is reshaping the field of physics, providing new ways to model complex systems, enhance predictive capabilities, and drive experimentation to unprecedented levels. The collaboration between AI and physics is not just an emerging trend but a paradigm shift that is accelerating discoveries across multiple domains.
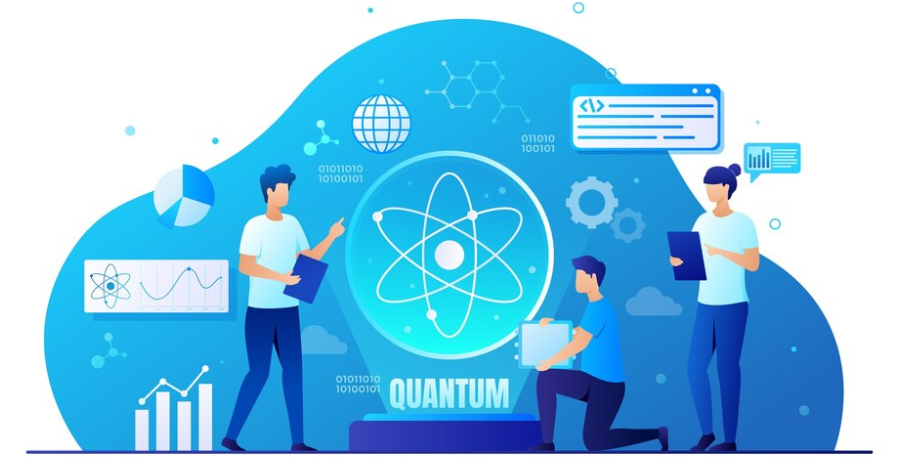
From quantum mechanics to astrophysics, machine learning algorithms are helping researchers analyze vast amounts of data, identify patterns, and even propose new theories. AI is bridging the gap between theoretical physics and experimental validation, making it a crucial tool for the future of scientific advancement.
The Evolution of AI in Physics
The integration of AI in physics is not a sudden revolution but rather an evolution that began decades ago. Early efforts saw the use of computational models to simulate physical phenomena, but these approaches were often limited by processing power and algorithmic efficiency. As machine learning techniques matured, physicists began incorporating AI-driven tools to enhance simulations and automate data analysis.
Breakthroughs in neural networks, deep learning, and reinforcement learning have enabled AI to process vast datasets that were once too complex for traditional methods. In recent years, high-energy physics experiments like those at CERN have relied on AI to sift through massive amounts of particle collision data. Likewise, AI has been instrumental in refining climate models and optimizing the design of fusion reactors. The increasing sophistication of AI has allowed it to move beyond mere data processing and into the realm of discovery, where it aids in formulating new theories and making novel predictions. Explore https://rescale.com/platform/ai-physics/ for more information on this fascinating topic.
AI in Physics Modeling
Modeling is a cornerstone of physics, providing a framework for understanding and predicting natural phenomena. AI has significantly improved the accuracy and efficiency of these models by refining computational physics techniques. In areas like quantum mechanics, AI-driven algorithms are being used to simulate quantum states and predict material properties with unprecedented precision.
One of the most promising applications of AI in physics modeling is in condensed matter physics, where deep learning techniques help uncover new phases of matter. Neural networks can process complex wave functions and identify hidden correlations that would be difficult to detect using conventional methods. Similarly, AI is assisting in the modeling of black holes, neutron stars, and galaxy formations, where traditional equations struggle with the sheer scale and complexity of calculations.
AI-enhanced modeling extends beyond theoretical physics into practical applications. In material science, AI-powered simulations accelerate the discovery of new materials with desirable properties for energy storage, semiconductors, and superconductors. These advancements reduce the time required to transition from theoretical designs to real-world applications, making AI an indispensable tool in physics research.
AI for Prediction in Physics
Prediction is another critical domain where AI is making a profound impact. One of the most well-known applications is in climate science, where AI models enhance weather forecasting by analyzing massive datasets and identifying patterns that elude conventional methods. AI-driven climate models provide more accurate long-term predictions and help policymakers make informed decisions about environmental challenges.
In particle physics, AI plays a vital role in predicting the outcomes of high-energy collisions. The Large Hadron Collider (LHC) generates petabytes of data from experiments, and AI algorithms sift through this information to identify potential new particles. By recognizing subtle anomalies in collision data, AI is helping scientists uncover evidence of physics beyond the Standard Model.
AIās predictive power is also transforming theoretical physics. Machine learning algorithms can analyze equations and identify possible solutions that physicists might overlook. In some cases, AI has been used to derive equations governing complex physical systems, such as fluid dynamics and plasma behavior. This capability allows physicists to explore uncharted territories, testing hypotheses, and refining theories with greater efficiency than ever before.
AI in Experimental Physics
Experimentation is a fundamental aspect of physics, and AI is revolutionizing the way experiments are conducted and analyzed. The rise of autonomous laboratories, where AI oversees experimental design, execution, and analysis, is changing the landscape of scientific research. AI-driven automation reduces human error, increases efficiency, and enables rapid iteration of experiments.
In fields such as astronomy and particle physics, AI-assisted image recognition is playing a pivotal role. Telescopes equipped with AI algorithms can detect exoplanets, identify cosmic phenomena, and analyze vast celestial datasets in real time. Similarly, AI is used in particle accelerators to optimize beam alignment and detect rare events that might indicate the presence of new particles.
The integration of robotics and AI is further enhancing laboratory automation. In experimental physics, robots equipped with AI-driven decision-making capabilities can adjust experimental parameters in real time, responding dynamically to unexpected outcomes. This adaptability accelerates the discovery process and enables researchers to explore a broader range of experimental conditions with minimal human intervention.
Conclusion
AI is redefining the way physics is studied, modeled, and tested. From enhancing simulations to driving discoveries, AI is unlocking possibilities that were previously unattainable. Its role in predictive modeling is reshaping climate science, high-energy physics, and fundamental theoretical research. In experimental physics, AI-driven automation and data analysis are accelerating discoveries while reducing human error.