Data tampering presents a risk to artificial intelligence (AI), raising concerns for developers as it continues to grow in prominence. The act of data poisoning entails the introduction of deceptive information into training data sets, which has the potential to compromise AI models. These compromised models might generate detrimental results, thereby impacting the trustworthiness of AI technologies.
AI programmers need to acknowledge the nature and significant consequences of data tampering incidents in AI systems. This is done by highlighting the importance of comprehending the workings of data poisoning attacks and taking necessary measures to minimize their adverse effects on system security and integrity.
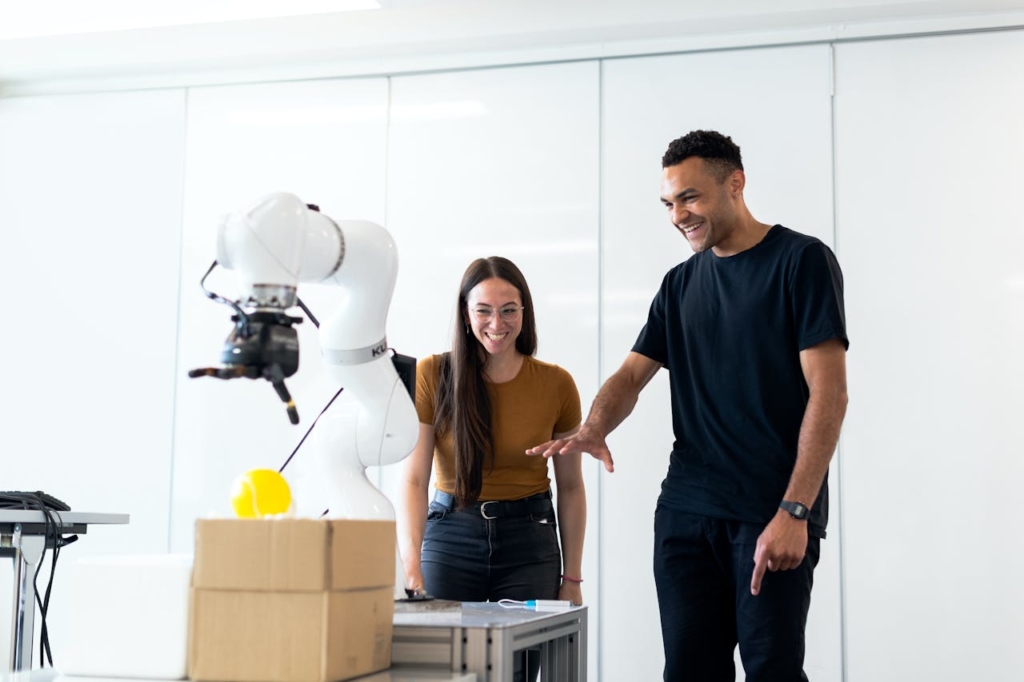
Varieties of Data Contamination
There exist types of data poisoning tactics that have consequences associated with them. One frequently encountered tactic involves label switching, where the perpetrator alters the labels of data points. This causes the AI system to acquire connections, resulting in false predictions.
Another type of attack includes backdoor infiltration tactics, where perpetrators implant a signal within the information that can lead to faulty behavior by the AI system once it is activated.
Effects on Artificial Intelligence Systems
Data contamination can greatly impact the effectiveness and trustworthiness of AI systems. If models are trained using data sets, they may generate results leading to potentially harmful outcomes. For example, in healthcare, inaccurate forecasts could result in diagnoses or treatment strategies that put patients at risk.
Ways to Identify and Stop Potential Issues
It can be quite a challenge to detect data poisoning; however, there are methods to recognize and address these risks effectively. One method is to keep an eye on the training process for any irregularities; unexpected patterns or deviations from the norm could be signs of data poisoning.
One approach involves data validation—an examination of the accuracy and reliability of training datasets to minimize the chance of contamination incidents. Certain methods like cross-outlier detection can pinpoint problematic data entries.
Teamwork
To tackle data poisoning effectively, teamwork between AI developers and researchers in collaboration with industry stakeholders is required. By sharing insights on risks and recommended strategies , a united front can be established to combat data poisoning together. Participating in industry events such as conferences, workshops, and online discussions presents chances for sharing knowledge and expertise.
Education plays a major role in addressing data contamination issues, as developers need to stay updated with the advancements and practices in AI security to combat emerging challenges effectively through ongoing learning and professional growth.
The Function of Regulatory Authorities
Regulatory agencies also play a part in combatting data contamination by setting norms and recommendations for the advancement of AI, which can encourage practices and bolster system security measures. Rules requiring openness and responsibility in AI systems can deter individuals and build confidence among users.
Adhering to mandates often motivates businesses to implement security protocols in place and showcase their dedication to creating secure and trustworthy AI systems by following established industry norms.
Upcoming Avenues for Exploration and Studies
As AI technology advances further in its development, it also means that the strategies employed to protect data will progress in sophistication. It is crucial to conduct research to outsmart these threats. Creating methods to identify and thwart data tampering will play a role in upholding the reliability of AI systems.
Delving into the application of algorithms and machine learning methods to detect data shows great potential for research advancement. It is beneficial to allocate resources towards AI security investigations as they can pave the way for solutions that bolster system strength.
Conclusion
Data manipulation continues to be a worry for developers working in AI because of its ability to undermine the trustworthiness and security of AI systems. It is crucial to grasp the manifestations of data manipulation and its consequences to create methods for identifying and preventing it. Working together with others in the field through education and regulatory measures plays a role in combating data manipulation. By keeping up to date and taking initiative in their work, developers in the field of AI can create systems that are secure and reliable, all while making sure that the advantages of AI technology are fully utilized without sacrificing safety or honesty.