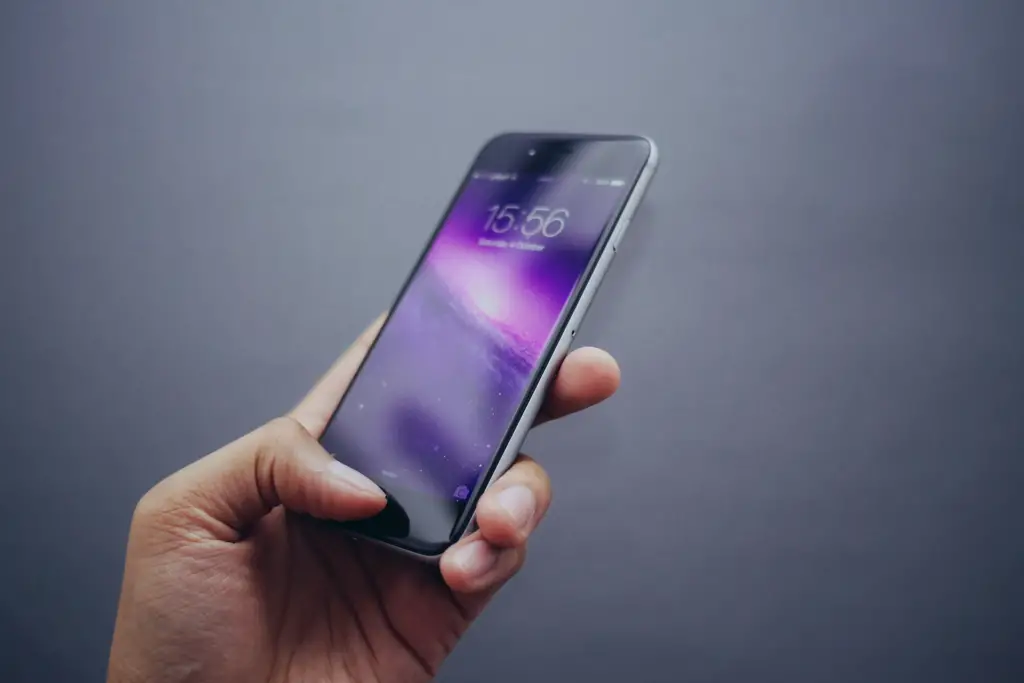
Introduction
For many, discussions surrounding facial recognition technology may seem a bit old hat. Given that the technology has seen wide adoption by Silicon Valley tech giants, banks, and governments for a variety of authentication needs, the technology is seen as matured. However, with the increased adoption of face detection comes the increased risk of attacks looking to subvert the technology.
Presentation Attacks
As the industry grows, the facial recognition market is expected to top over 12 billion USD by 2027, the need for criminals to subvert the technology will grow. Security researchers have come across several attacks on the technology by hackers and other cybercriminal groups that can be broadly placed into two categories of attack: presentation attacks and indirect attacks. Presentation attacks look to target biometric vulnerabilities.
This typically involves a threat actor attempting to trick the sensor and supporting software into the technology verifying the threat actor as the individual impersonated. This has been done in the past using a photo, a mask, a synthetically reproduced fingerprint, or a copy of the iris. In terms of exploiting facial recognition sensors photographs, video, and 3D printed masks of the individual have been used to try and impersonate an individual.
Indirect Attacks
Rather than attempting to trick the sensor, indirect attacks attempt to compromise the interior of the system. The interior can be seen as the software and databases used by the system to confirm an individualās identity once data is captured from the system. Compromising the interior often involves the use of malware or other tactics traditional cybersecurity threats will attempt to use. In general, these can be defended against using the same security hygiene methods that most organizations should have in place already.
Defending against Presentation Attacks
When tech giants like Apple adopted several face detection applications, users applauded the ready adoption of such technology. Unfortunately, hackers were already at work looking to subvert the technology and find vulnerabilities they could exploit. Fortunately, security researchers were developing methods to prevent exploitation.
Many of these methods became known as anti-spoofing and typically involved developing countermeasures to stop sensors from being tricked. This can be done in a variety of ways including developing sensors to detect signs that what has been detected is alive. These methods will typically prevent the attacker from simply using a printed image or video of the impersonated individual.
Other countermeasures include upgrading hardware with a 3D camera, which can also help detect liveness as 2D images used by the attacker will fail to be verified, preventing the attack. Installing a 3D camera is not always possible, in those instances, challenge-response methods can be employed. These typically require the person identifying themselves to smile or move their head in a certain way which would help prevent a printed mask from successfully subverting the system.
Deep Learning
Another method of defense that will see increased adoption in the years to come is deep learning algorithms. The algorithms can effectively learn from a variety of data inputs how to detect if the system is under attack. As these develop specific traits of individuals can be incorporated into the database making impersonation nearly impossible.
Conclusion
Facial recognition technology is reaching a level of maturity and acceptance few would have predicted at its inception. This maturity arose in part due to talented security researchers discovering holes to exploit and fill them with intelligent solutions preventing attackers from using the technology for malicious purposes.